Bearing failure forecast prevents loss of 53 MWh and reduces critical temperature by 15°C
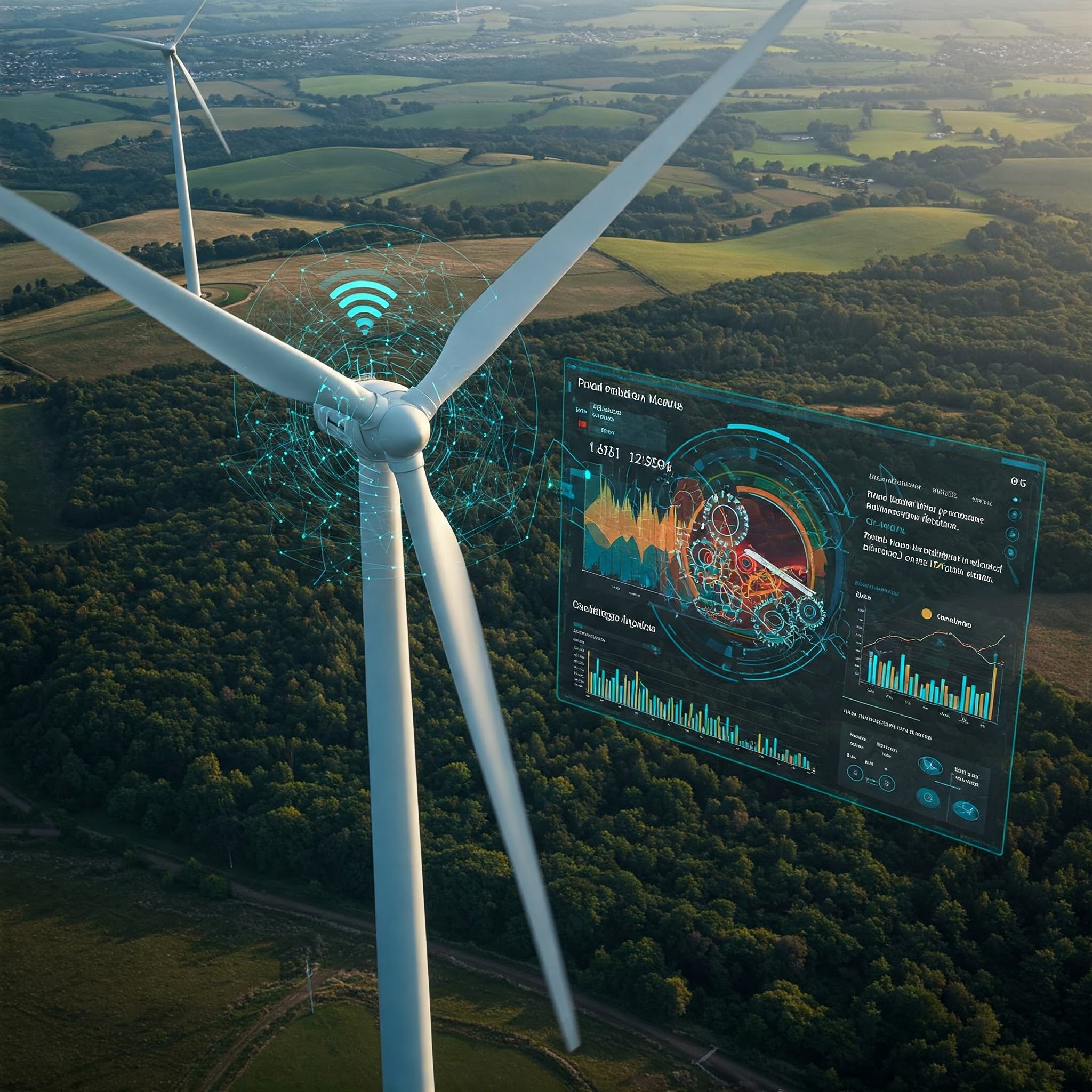
During continuous monitoring of the wind farm located in the Northeast, a GE 1.7-100 turbine (WTG 09) began to exhibit anomalous thermal behavior starting on 09/12/2024. Delfos identified, through a predictive alarm, a progressive temperature increase in the main rotor bearing, one of the turbine’s most critical components.
The initial analysis showed that:
- The turbine had not yet been mapped for this failure in the client’s system.
- The behavior was subtle and gradual, making it difficult to identify without a machine learning-based tool.
- The component operated at higher-than-expected temperatures for the same power regime, indicating growing thermal inefficiency.
This early detection was only possible due to Delfos’ machine learning-based approach, using individualized turbine behavior history and cross-referencing time-series data with intermittent anomalies.
Implemented Solution
1. Detection with the Prediction Module
Delfos’ Prediction module, trained with 10-minute time-series data over 12 months, detected a consistent temperature deviation in the main rotor bearing. This module compares the current machine behavior with expected patterns, taking into account variables such as:
- Generated power
- Ambient temperature
- Operational regime
- Typical heating curves under different conditions
This type of analysis allows anomalies to be identified even when there is no direct exceeding of fixed thresholds, as with traditional systems based solely on setpoints.

Figure 1 – Baseline temperature and start of gradual heating trend.

Figure 2 – Exceeding 60°C and increasing trend not correlated with power.
2. Corroboration with Alarms and Events
Between 12/12/2024 and 01/11/2025, the turbine began to exhibit intermittent stoppages due to automatic triggering by the SCADA system, which halted operations upon reaching the 65°C setpoint. In this case, Delfos’ system was activated when the temperature rise was already advanced, indicating that the alarms could have been avoided if predictive monitoring had been active from the beginning of the anomalous behavior.
Delfos consolidated evidence showing:
- Correlation between thermal peaks and stoppage events, although these already reflected an advanced stage of failure
- Accumulated productivity reduction
- History of repeated "shaft bearing over temperature" alarms
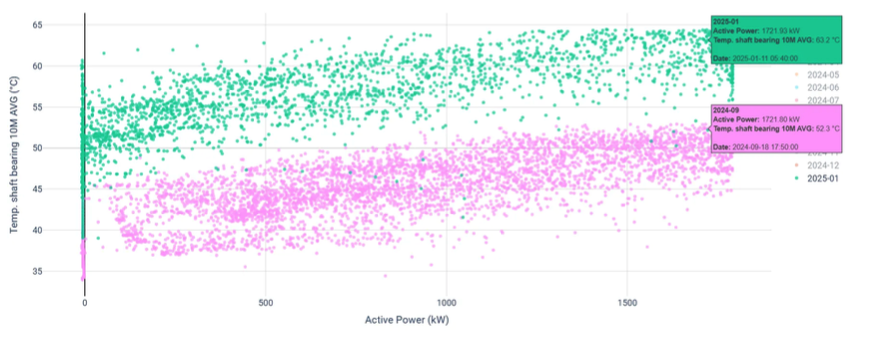
Figure 3 – Record of stoppages and correlation with setpoint.

Figure 4 – Comparison with neighboring turbines: +15°C difference.
3. Advanced Comparative Analysis
To reinforce the evidence, Delfos applied a comparative analysis between machines in the same wind farm — an approach that detects relative performance deviations. WTG 09’s bearing operated up to 15°C above the other turbines under similar operational conditions.
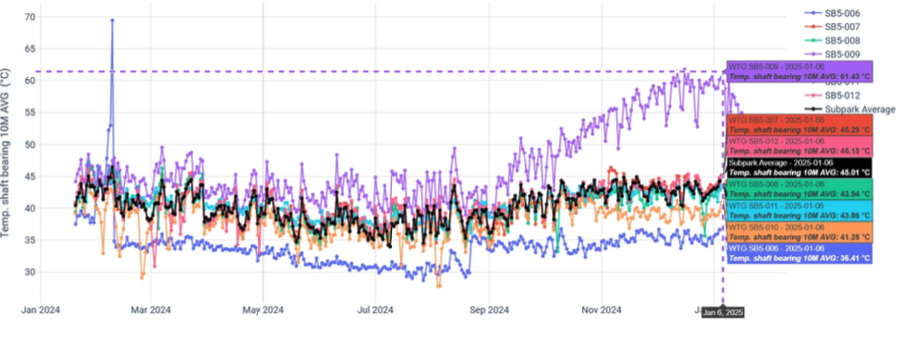
Figure 5 – Temperature dispersion visualization between turbines highlighting the outlier.
Results Achieved
After the formal report issued by Delfos’ Performance Engineering team, a technical inspection and subsequent replacement of the main rotor bearing were carried out on 02/15/2025.
Observed changes post-intervention:
- Immediate reduction of average temperature by 15°C
- Complete cessation of thermal alarms after 01/28/2025
- Continuous thermal stabilization, confirmed by updates through 04/14/2025
- Thermal behavior returned to align with the expected profile for turbines under nominal regime
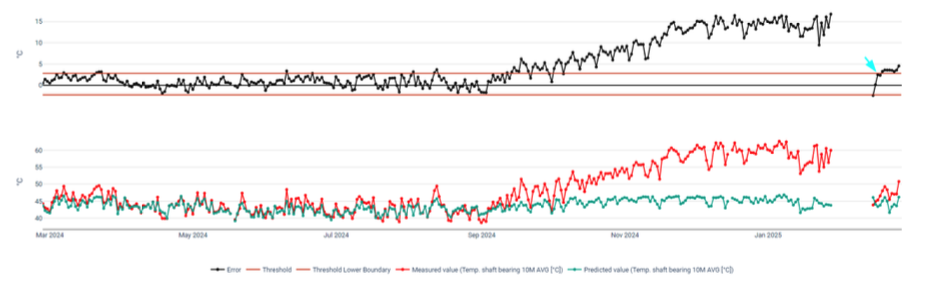
Figure 6 – Post-maintenance thermal drop.
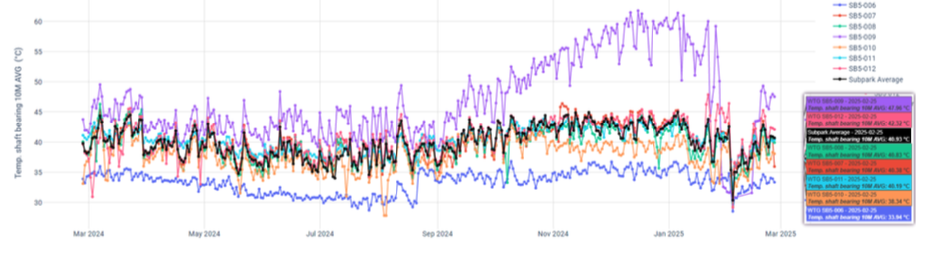
Figure 7 – New stable thermal curve.
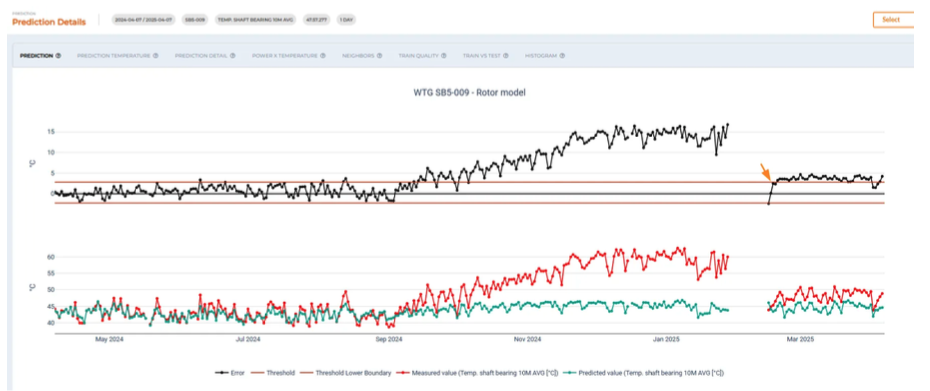
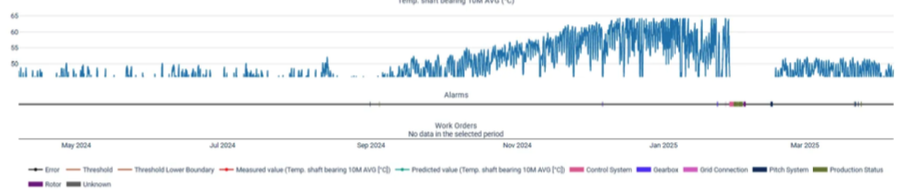
Figures 8 and 9 – Two-year historical comparison: normalized behavior.
Energy impact:
- The turbine was stopped for approximately 40 hours due to alarms before the intervention.
- The accumulated unproduced energy was estimated at 53 MWh.
- Preventive maintenance avoided further losses and possibly damage to the rotor shaft itself, whose repair would be significantly more expensive.



Figures 10, 11, 12 – Record of stoppages and unproduced energy.
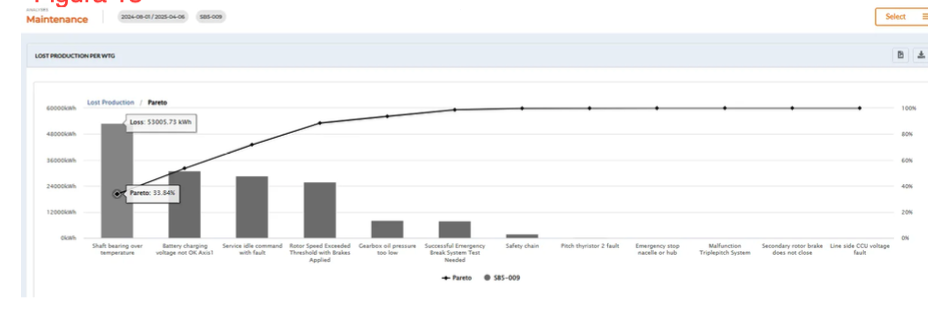
Figure 13 – Final cessation of alarms.
Conclusion
This case clearly demonstrates the value of AI-assisted predictive maintenance. Delfos’ early diagnosis enabled a proactive response, preventing the progression of a critical failure and ensuring:
- Energy recovery
- Greater turbine availability
- Reduced risk of catastrophic failure
- Potential cost savings from anticipating corrective maintenance
Delfos’ role goes beyond monitoring: it is applied intelligence for proactive decision-making, continuously integrating engineering, data, and performance.
Big Numbers
- 15°C temperature reduction in the bearing after maintenance
- 53 MWh of unproduced energy avoided
- 40 hours of unavailability mitigated
- Failure identified before any internal mapping
- 53% reduction in thermal difference between machines
Book a meeting
Let's connect and forge new partnerships
Custom Renewable Energy Solutions
Contact us today to discuss your renewable energy needs and find the perfect solution for your business.